Watershed
Include <mln/morpho/watershed.hpp>
-
template<class Label_t, class I, class N>
image_ch_value_t<I, Label_t> watershed(const Image<I> &ima, const Neighborhood<N> &nbh, int &nlabel, bool waterline = true) Watershed by immersion as defined in [BM92]. The catchment basins are labeled from 1 to n, and the special label 0 is used for watershed lines.
- Template Parameters:
Label_t – The type of label (must be signed
Integral
)- Parameters:
input – Input image
nbh – Neighborhood considered
nlabel (out) – Number of catchment basins
waterline – Add the watershed lines in the resulting segmentation
- Returns:
A labelized image
- Exception:
N/A
-
template<class Label_t, class I, class N, class S>
image_ch_value_t<I, Label_t> watershed_from_markers(I &&input, N &&nbh, S &&seeds, int &nlabel) Watershed by immersion as defined in [BM92] with given markers. A marker is one pixel on the seed image which is greater than 0.
- Template Parameters:
Label_t – The type of label (must be signed
Integral
) and the maximum value of this type must be higher or equal to the maximum value of the markers image value type.- Parameters:
input – Input image
nbh – The considered neighborhood
seeds – An image with markers. The markers label should be greater than 0.
nlabel (out) – The number of catchment basins
- Returns:
A labelized image
Notes
Complexity
The algorithm is quasi-linear and requires \(n\) extra-memory space.
References
Example 1: Cell segmentation
The distance transform is performed. Maxima correspond to cell centers. A dilation by a small disc removes the non-meaningfull maxima.
Invertion of the distance image so that maxima become minima
Watershed segmentation
Input labelization w.r.t in segmentation labels
// (1) Compute the distance transform
mln::se::wmask2d weights = {{3, 2, 3}, //
{2, 0, 2},
{3, 2, 3}};
auto d = mln::labeling::chamfer_distance_transform<uint8_t>(input, weights);
// Remove non-meaninfull extrema
d = mln::morpho::dilation(d, mln::se::disc(5));
// (2) Inverse the distance
auto dinv = mln::transform(d, [](uint8_t x) -> uint8_t { return UINT8_MAX - x; });
// (3) Run the watershed segmentation
int nlabel;
auto ws = mln::morpho::watershed<int16_t>(dinv, mln::c8, nlabel);
// (4) Labelize input
auto output = mln::view::ifelse(ws == 0, 1, mln::view::ifelse(input, ws, 0));
![]() |
![]() |
Input image (note that blobs may touch) |
Distance transform and dilation (after heat LUT) |
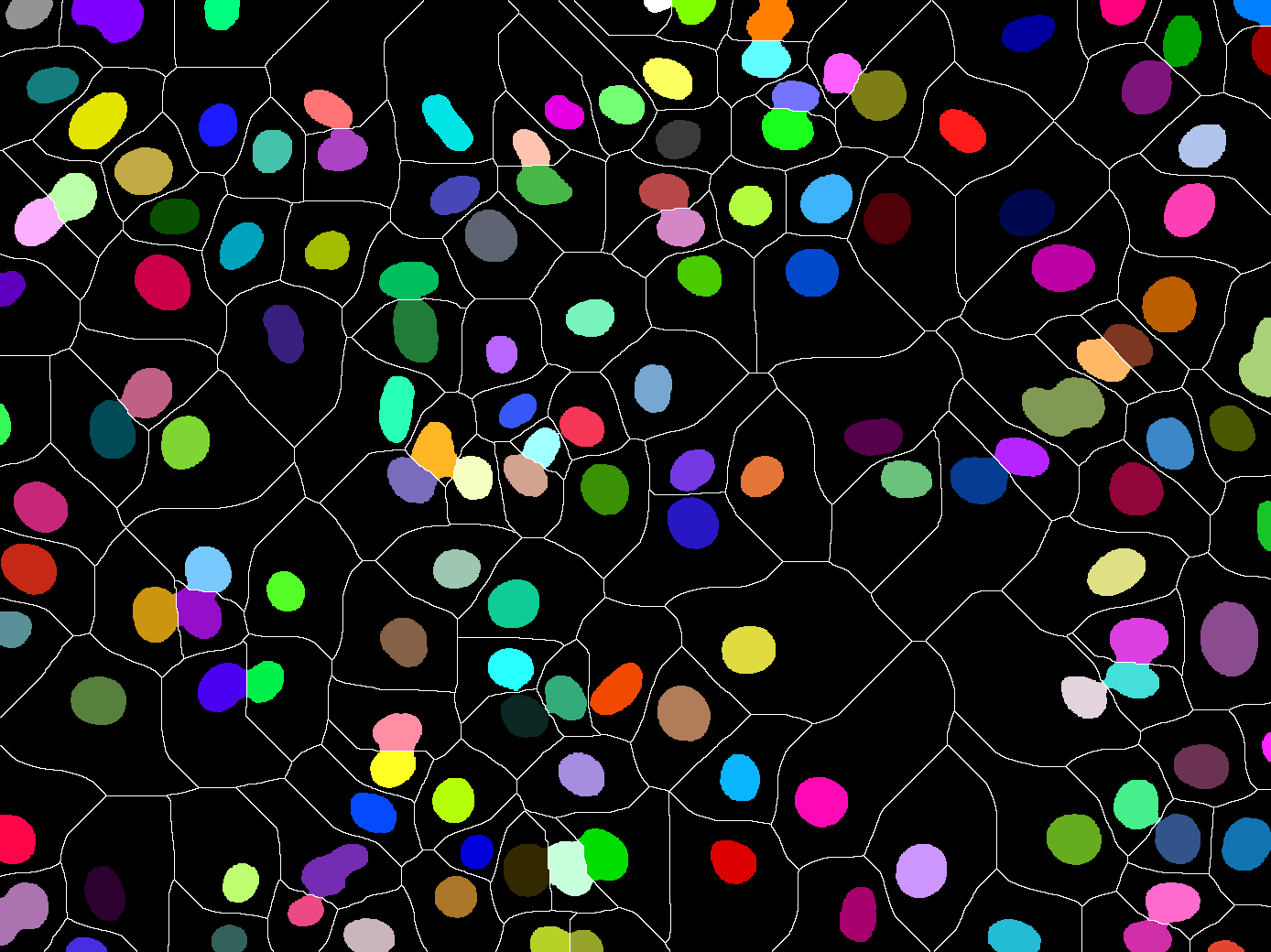
Segmented blobs and watershed lines (labels displayed in false colors).
Example 2: Watershed from markers
This example demonstrates how to use the watershed based on input markers.
// (1) Get input image and markers image
mln::image2d<std::uint8_t> input = ...;
mln::image2d<std::uint16_t> seeds = ...;
// (2) Compute the Beucher gradient
auto grad = mln::morpho::gradient(input, mln::se::disc(3));
// (3) Compute the watershed
auto out = mln::morpho::watershed_from_markers<std::int32_t>(grad, mln::c8, seeds, nlbl);
![]() |
![]() |
Input image (with markers in red) |
Segmented image with watershed lines (labels are displayed in false colors). |